Nicholson Price is a professor of law at Michigan Law. He teaches and writes in the areas of intellectual property, health law, and regulation, particularly focusing on the law surrounding innovation in the life sciences. He is a core partner at the University of Copenhagen’s Center for Advanced Studies in Biomedical Innovation Law and co-principal investigator of the Project on Precision Medicine, Artificial Intelligence, and the Law.
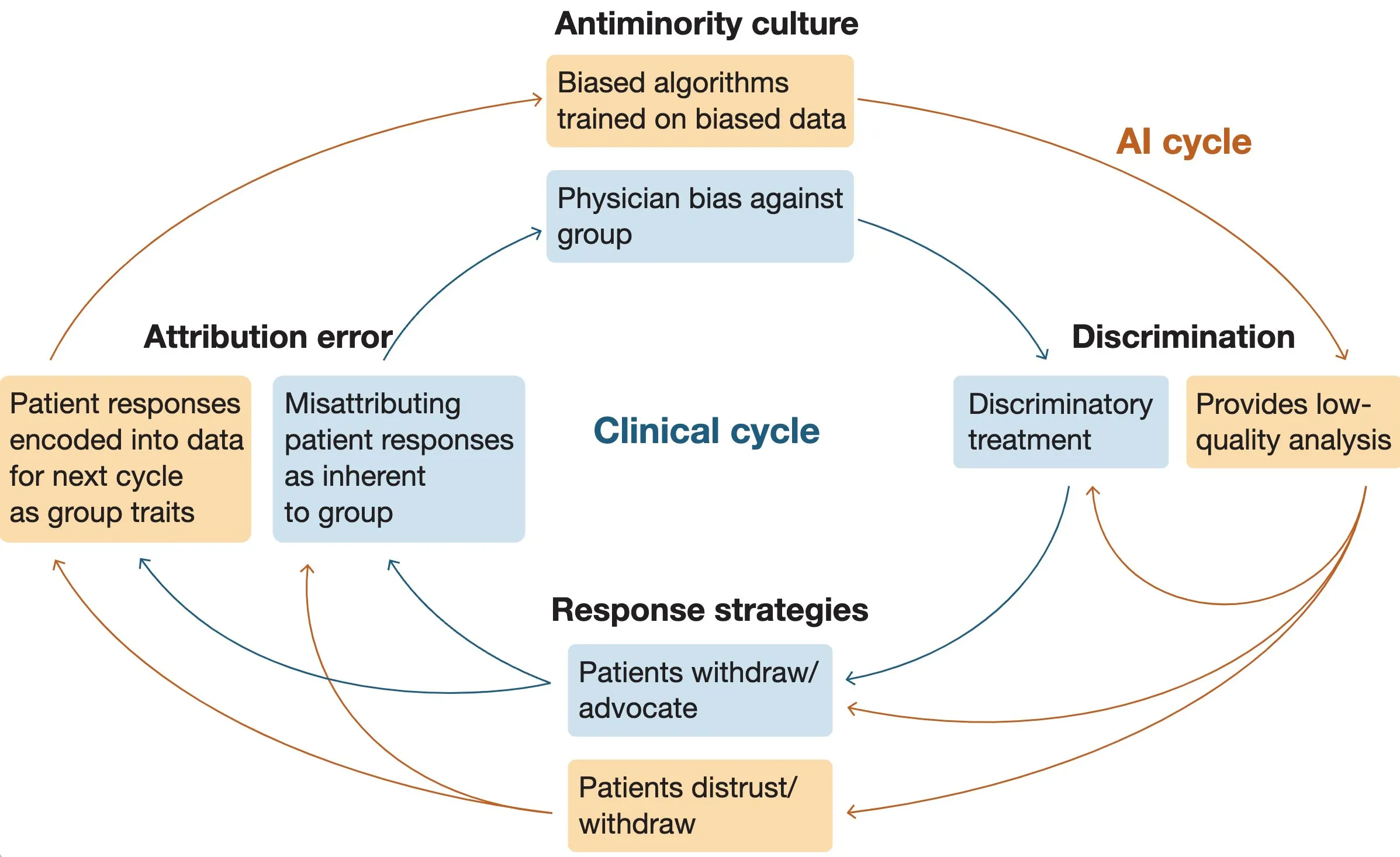
He authored “Exclusion Cycles: Reinforcing disparities in medicine” in the September 8, 2022, issue of Science. His co-authors are Professor Ana Bracic of Michigan State University and Professor Shawneequa Callier from the George Washington University School of Medicine and Health Sciences.
Below, he answers five questions about why he wrote the article and why it matters. Professor Bracic also responds to one of the questions based on her research.
1. Your work draws on a theory of “exclusion cycles.” Can you explain it?
The exclusion cycle theory helps explain why exclusion of some groups is so persistent and hard to fix. Basically, the cycle has four parts: (1) anti-minority culture, which leads to (2) discrimination by dominant groups against minoritized groups; (3) response strategies by the minoritized groups to that discrimination (like withdrawal or resistance); and (4) the attribution error, where dominant groups attribute those response strategies to inherent characteristics of the minoritized group, rather than to the discrimination.
That attribution error feeds back into anti-minority culture, and the cycle repeats and gets stronger. Professor Ana Bracic, a co-author on this paper and an assistant professor of political science at Michigan State, developed the theory in a 2020 book called Breaking the Exclusion Cycle, based on extensive empirical work studying Roma/non-Roma relations in Europe.
2. These cycles are part of a larger social dynamic. How do they apply to medicine?
We see the exclusion cycle throughout clinical practice, and it helps explain why exclusion is so intractable in medicine, too. In the paper, we draw on the example of Black patients and pain, where the individual pieces of the cycle have been well studied.
Empirical studies show that many medical providers believe that Black patients are biologically different from white patients and feel pain less severely (anti-minority culture); as a result, physicians are more likely to provide lower doses of pain treatment (discrimination). Black patients may justifiably withdraw from treatment relationships (response strategies), and physicians may attribute that withdrawal to characteristics of the patients themselves (“Black patients are bad patients” rather than “that patient reasonably responded to discriminatory treatment”)—leading to stronger anti-minority culture.
The pieces of this cycle are well known by now, but we think putting them together helps us better understand the whole picture.
3. How do artificial intelligence (AI) and big data make matters worse?
This is the tricky bit! Adding artificial intelligence to the picture threatens to entrench exclusion cycles even more. AI can follow basically the same type of exclusion cycle, even though AI can’t think and can’t be deliberately racist.
AI, however, is trained on data from existing medical practice, which has bias and discrimination embedded throughout, so AI learns from bias (anti-minority culture). The AI systems then are likely to make poor recommendations for minoritized patients (discrimination). And the patients are—justifiably!—likely to resist AI recommendations or the use of AI when the system gives them bad advice or leads to bad outcomes (response strategies). When the outcomes aren’t as good, because predictions were bad and because patients might have resisted them, the AI learns bad lessons from these new data and is likely to associate them with patient characteristics rather than realizing that it all resulted from the earlier discrimination (attribution error). And that feeds back into the next cycle, reinforcing bias going forward.
But actually, it’s even worse, because the AI and the clinical practices feed into each other and reinforce each other. For instance, AI discrimination (bad predictions) will look like physician discrimination to patients, even if the physicians aren’t themselves biased at all, providing energy to the clinical cycle. And bad predictions may result in patients withdrawing not just from the use of AI, but from the physician relationship entirely. The two cycles can get very difficult to disentangle, and tackling them separately runs into real problems.
4. You refer to “minoritized” patients in your paper. How do they differ from minorities?
We use “minoritized” instead of “minority” in part because it recognizes that minorities are constructed groups. In fact, in particular instances, a group that is treated like a minority might not be a numerical minority (e.g., Black South Africans). It helps flag that the minority/majority isn’t a simple question of numbers; it’s about how members of different groups think about and treat each other.
5. How do you approach this problem from (a) a legal perspective and (b) a diversity/research perspective to make matters more inclusive?
Nicholson Price: From a legal perspective, I think about what levers law and policy can use to help improve the situation. For instance, can FDA regulation of AI systems as medical devices include requirements of representativeness in the underlying data to help combat bias? (Even if it can, what about AI systems that FDA doesn’t see?) Can legal liability attach for discrimination of the kinds we talk about? Could grant funding help build the infrastructure for more equitable care, data collection, and AI in smaller health care settings?
From a diversity/research perspective, I think about trying to look at these really complex problems from different directions with a focus on justice, equity, diversity, and inclusion. That’s part of the reason this project has been so exciting for me; there’s no way we could have pulled this together without insights from law/policy (that’s me), the framing from social sciences (that’s Professor Bracic), and expertise in bias in medicine and medical big data (that’s Professor Callier).
Ana Bracic: From a diversity/research perspective, I think about interventions that might help us break these cycles. On the clinical side, placing minoritized physicians and data scientists on care and research teams is an effective potential intervention, as is education on systemic biases more generally. On the AI side, carefully evaluating performance data in the process of retraining might help us ensure that new biases about patients are not incorporated into the system. Importantly, because the clinical and the AI cycles feed into each other, the interventions would have to be carried out together to have a shot at fully breaking the dynamic.